Alexis is a learning experience designer. Well, last week she was in an instructional design role but with her department’s rebranding that title changed this week. Anyway, her client handed her a pile of content, the name and email of the person who created the content, and asked for eLearning that is engaging and produces specific, desired changes in employees’ workplace performance. She will need to come up with an eLearning design that will deliver the desired performance. If only there were easy-to-use resources on how to get this started....
There’s so much change in the eLearning industry. eLearning authoring software such as Camtasia, Articulate, and Captivate is constantly updated to fit with evolving demands for beautiful and functional courses. eLearning lingo changes often, too. From clients who used to request reusable learning objects to those who want MOOCs, blended learning, scenarios, storyboards, rapid prototypes, microlearning, nanolearning, augmented reality, virtual reality, and who knows what’s next, there’s a lot to keep up with!
Where does one start? Currently, most people who design and develop eLearning objects think of them as instructional environments that are delivered to learners on internet-connected devices such as mobile phones, tablets, and computers. Some think eLearning is a webinar or a course in an LMS that an instructor leads and gives custom feedback to learners’ deliverables. Others might think of eLearning objects as being housed anywhere such as in email, in SMS messages, on YouTube, on specialized devices (e.g., Apple Fitbit, Leapfrog, V-tech) where it’s self-paced and interactive, usually integrated with automated feedback systems. We will focus specifically on eLearning object design approaches for self-paced components of an asynchronous or blended delivery context of any length. These types of eLearning objects often appear as videos, asynchronous courses, apps, tutorials, job aids, and interactive performance support systems.
So let’s make things a little easier by focusing on what will (almost certainly) never change—basing your first instructional or learning design decisions for any given eLearning object on sound principles. In this article we provide a set of time-tested instructional design approaches grounded in research and evidence-based practice to guide your first important design decisions. No matter the shelf-life desired, formatting, length, or what technology comes out next, using these design approaches will deliver desired results.
How do you choose an instructional design approach to get the desired performance results?
Complete a performance context analysis
Before designing an eLearning module, you need to know more about the project and audience. We recommend starting with a performance context analysis. This is when you begin to figure out who needs to do what and where they need to do it and why it’s important now. The “who” is important to consider first, to ensure you can design a solution that meets the needs of the learners. You also should know what these learners need to do and where they need to do it so you can provide contextualized support that will help them perform to desired expectations. For example, being able to describe the components of a storyboard won’t necessarily help you design an effective eLearning experience for employees who work in Mexico at a car manufacturer.
If you know why it’s important to design the eLearning object now, you will be able to build something that’s aligned with the strategic goals, something shorter if it’s only for reinforcement, or something more robust for new information. You can ask your client questions like:
- Who is this for?
- What motivates these learners?
- What prompted this need?
- Is this new information, or is this reinforcement of what they should already know and be able to do, or both?
- How does this align with our strategic goals?”
- What would you like to see the learners do with this information on the job?
Determine the performance outcome statement(s)
This performance context analysis should give you enough information to be able to draft one or more performance outcome statements. Performance-based outcomes that are clear will help you build your design and may also help your learners understand what’s expected of them. The statement(s) should describe the desired observable behaviors on the job that learners should be able to perform long after they complete the eLearning course.
Performance outcomes should be observable and measurable—that is, they describe the specific performance you want to see someone do. They should also describe the behaviors most typically expected while on the job, long after the course is over. They should be specific enough that an evaluator could reliably assign a score based on multiple performance criteria during any given observation using a performance checklist instrument, or if absolutely necessary, then a knowledge check quiz answer key.
For example, the outcome “the learner can wash a car” for a new boutique, high-end car wash employee does not tell the learner what they need to do in order to meet or exceed expectations of a line manager or evaluator. Different line managers or evaluators would likely give different scores and feedback based on different criteria. Instead, give direct, conditional objectives so your learner knows exactly what they need to do and your line managers and evaluators encourage performance that meets a standard criterion. For example, “The learner scrubs the entire exterior of each car including mirrors and rims, given wash formula and large sponge for 10 minutes.”
Writing clear, direct outcomes frames the entire learning experience. They help keep you—the designer—from overwhelming the learner with too much information at one time. Ever been to a seminar or lecture that left you exhausted and wondering what the purpose of it was? Yeah, we are trying to avoid that.
Organize one or more subordinate statements under an overarching performance outcome
If you realize that you have more than one performance outcome statement drafted you can begin to intuitively decide whether the other statements fit as a learning objective under one or more of the performance outcome statements. Once you have organized these statements into a first draft hierarchy, you can work on the content analysis for each overarching performance outcome, one at a time.
You might realize now that you need multiple learning objectives to scaffold learners towards a larger performance outcome. You can use these smaller learning objectives to ensure content stays relevant to the higher performance outcome and helps to chunk the lesson content into smaller components. These smaller learning objectives can help you sequence instructional units or lessons that take learners from getting started to fully performing on the job. Now, let’s dig into content analysis.
Conduct a content analysis for each performance outcome statement
Once you know the performance context and performance outcome, you will be able to complete a content analysis. This is when you begin to identify the different chunks of related content and the types of these chunks that you already have access to, or the chunks and types of content you might need to get access to. It’s important to determine the types of content because then you can select an overarching design approach that will deliver to your clients’ desired results.
Determine knowledge type: declarative, procedural, situated
There are three main types of knowledge, as shown in Figure 1: declarative, procedural, and situated (Chyung, 2007). Each type becomes progressively more difficult or complicated to attain than the former.
Declarative knowledge is described as knowing what something is. When you learned how to speak, you likely pointed at things and said what they were: “apple,” “Da-Da,” “puppy”. Eventually, you realized that people could understand you better if you stringed these words together: “Socks on,” “Up on Ma-Ma.” This type of learning is called procedural knowledge, described as knowing how to do something that follows a general pattern. Later, you formed original sentences based on the specifics of any given nuanced situation. This is called situated knowledge, described as knowing when, where, and why, to do something: “Select a retirement savings strategy”, “Give performance feedback to a direct report.”
Figure 1: Knowledge types from most basic to most advanced
As eLearning designers, it is our responsibility to determine the knowledge type(s) needed and the level of proficiency of our audience. Sometimes our audience has a baseline of knowledge. Consider automobile line worker “Sheila” being promoted to manager. Sheila knows about the parts (i.e., declarative knowledge) and steps needed to assemble (i.e., procedural knowledge) the car. What Sheila doesn’t know how yet is how to troubleshoot or make decisions (i.e., situated knowledge) when something goes wrong. The eLearning designer should then create a situated knowledge eLearning design.
Organize knowledge type chunks into a sequence
You might be wondering how these categories, which range from most basic to most advanced, become useful. Now that you know what kind of knowledge types you will need to incorporate into your eLearning, becoming creative and effective with your instructional design approach gets easier. Often it’s important to follow a basic sequence of instruction so that learners can meet the performance expectations as quickly as possible (Merrill, 2018; Schalk et al., 2018). This usually starts with introducing declarative knowledge before procedural knowledge and saving situated knowledge for last in eLearning design in a tell-and-practice (TP) sequence (Merrill, 2018; Schalk et al., 2018).
If you need to introduce declarative knowledge, you can do so with basic instructional approaches and feedback. These instructional approaches might include lecture-type presentations such as matching or making selections. The feedback you would share could be formative, summative, immediate, self-regulation, or scaffolding, to note what’s correct and why, and/or what’s incorrect and why, and/or what to consider next, and/or where to review more information, (Willert, 2021). If you are aware that you need to help learners pick up new processes or procedures, you can focus your eLearning design on simulating an environment, or demonstrating how to engage with a process or move through it (Smith & Regan, 2004). And, scenario-based eLearning designs can introduce your learners to common scenarios or situations that require the nuanced decisions and the common consequences they would likely encounter as they learn to perform to expectations (Gavarkovs et al., 2019; Choi et al., 2020). This progression is depicted in Figure 2, below.
Figure 2: Common instructional design progression for different knowledge types
We’d like to caution you that we’ve encountered plenty of situations where it’s not required to learn extensive declarative knowledge before procedural knowledge or gain mastery of procedures before attempting to learn novel situated knowledge. In fact, sometimes, it may be better or more engaging to teach learners about a procedure first (e.g., what order of tasks one needs to go through and what buttons to click when operating a machine) before going deeper into specific declarative knowledge (e.g., knowing exact what each component in the machine is). Acquiring declarative knowledge supports the procedural knowledge and helps master the procedural knowledge, but it doesn't always have to be a prerequisite.
Similarly, sometimes learners can be introduced to a situated learning content where they learn when, where, and why types of information first (e.g., job harassment case studies), and then they continue to learn about specific declarative and procedural knowledge (e.g., what is and what is not harassment, how to file a complaint). In short, there are times when a problem-solving then instruction (PS-I) sequence can be superior to a traditional TP (Schalk et al., 2018).
Lastly, we’d like to emphasize that either sequence type can be started with sharing a story (e.g., contextualized experience, reflection, meaning or value, problem, or trigger event) to get learners interested and focused on the larger performance goal (Doolani et al., 2020) or desired cultural change (Rea, 2021).
Rarely will eLearning be created for one specific knowledge type. Most often there will be a performance outcome that requires learners to demonstrate command of both declarative knowledge and procedural knowledge types. Sometimes there will be multiple chunks of content associated with each knowledge type. Often enough, there will be a performance outcome that requires learners to demonstrate declarative knowledge, procedural knowledge, and situated knowledge. You can help learners achieve these larger performance outcomes by chunking eLearning course designs down into smaller modules with one to three learning objectives (Shipley, Stephen & Tawfik, 2018). We recommend creating a visual map of how mastery of each content chunk contributes to someone being able to meet the desired performance outcome, as shown in Figure 3 below.
Figure 3: Examples of knowledge types that range from most basic to most advance in relationship to a performance outcome in a visual map
Sequencing these modules, from the most basic declarative knowledge before introducing procedural knowledge, can help many of your learners to feel they are well-supported and able to be successful with the least amount of frustration possible. Once learners have a command of declarative and procedural knowledge, you can help them master situated knowledge—the most advanced knowledge type—if needed. One way to arrange a course related to a desired performance outcome is to create modules with related lesson topics.
Below in Figure 4 you will see this course will have two modules and eight lessons. It’s intended for a specific organization with specific audience needs to deploy a minimum viable product in a way where the instructional designer can achieve a significant return on investment. It’s not intended to be a catch-all course, so you will notice that there are only two main learning modules—each with one situated learning objective included. The performance outcome is focused on retention and related to a strategic organizational objective to decrease wasted resources.
Figure 4: Example lesson numbering patterns for modules 1 and 2
In this example, we don’t need to pursue one module before the other module because they are complimentary chunks. One does not necessarily build on the other, yet. However, researchers are finding improved outcomes when learners are able to build on prior knowledge or experience with new knowledge in a tell and practice sequence (Merrill, 2018; Schalk et al., 2018) that’s aligned with a larger task or problem-centered organizational performance goal in well-designed environments (Kraiger & Ford, 2021; Longo & Rajendran, 2021). Thus, we could design the training such that learners start with the declarative knowledge before jumping into the procedural knowledge associated with module 1 and 2. Then, we can build reinforcement of the declarative knowledge into lessons 2, 4, and 7.
Likewise, if learners gain mastery of the procedural knowledge before jumping into the situated knowledge in module 1 and 2, we can then reinforce what we shared previously. Similarly, if we require a module sequence, we could reinforce what students learned in the first module when we went to develop the second module and follow the numbering pattern for lessons in Figure 3.
In short
In summary, to get started with chunking and sequencing eLearning design, we recommend following a timeless, holistic (i.e., systemic and systematic), evidence-based practice approach. This approach includes the process of conducting a contextualized performance analysis, defining the larger performance outcomes aligned with strategic business objectives, organizing additional subordinate performance statements under one or more main outcomes, determining the knowledge types associated with each subordinate outcome, and then selecting a lesson sequence that’s appropriate for your audience. While a problem-solving then instruction (PS-I) sequence can be superior, a traditional TP sequence is often more efficient (Schalk et al., 2018). Either sequence type can be started with sharing a story (e.g., problem or trigger event) to get learners interested and focused on the larger performance goal (Doolani et al., 2020; Rea, 2021).
References
Choi, S., Yuen, H. M., Annan, R., Monroy-Valle, M., Pickup, T., Aduku, N. E. L. & Ashworth, A. (2020). Improved care and survival in severe malnutrition through eLearning. Archives of disease in childhood, 105(1), 32-39. Doi:10.1136
Chyung, Y. C. (2007). Learning object-based e-learning: content design, methods, and tools. Learning Solutions e-Magazine, August 27, 2007, 1-9. https://www.elearningguild.com/pdf/2/082707des-temp.pdf.
Doolani, S., Owens, L., Wessels, C. & Makedon, F. (2020). vis: An immersive virtual storytelling system for vocational training. Applied Sciences, 10(22), 8143.
Gavarkovs, A. G., Blunt, W. & Petrella, R. J. (2019). A protocol for designing online training to support the implementation of community-based interventions. Evaluation and Program Planning, 72(2019), 77-87.
Kraiger, K. & Ford, J. K. (2021). The science of workplace instruction: Learning and development applied to work. Annual Review of Organizational Psychology and Organizational Behavior, 8, 45-72.
Longo, L. & Rajendran, M. (2021, November). A Novel Parabolic Model of Instructional Efficiency Grounded on Ideal Mental Workload and Performance. International Symposium on Human Mental Workload: Models and Applications (pp. 11-36). Springer, Cham.
Merrill, M. D. (2018). Using the first principles of instruction to make instruction effective, efficient, and engaging. Foundations of learning and instructional design technology. BYU Open Textbook Network. https://open.byu.edu/lidtfoundations/using_the_first_principles_of_instruction
Rea, E. A. (2021). "Changing the Face of Technology": Storytelling as Intersectional Feminist Practice in Coding Organizations. Technical Communication, 68(4), 26-39.
Schalk, L., Schumacher, R., Barth, A. & Stern, E. (2018). When problem-solving followed by instruction is superior to the traditional tell-and-practice sequence. Journal of educational psychology, 110(4), 596.
Shipley, S. L., Stephen, J. S. & Tawfik, A. A. (2018). Revisiting the Historical Roots of Task Analysis in Instructional Design. TechTrends, 62(4), 319-320. https://doi.org/10.1007/s11528-018-0303-8
Smith, P. L. & Ragan, T. J. (2004). Instructional design 3rd Ed. John Wiley & Sons.
Willert, N. (2021). A Systematic Literature Review of Gameful Feedback in Computer Science Education. International Journal of Information and Education Technology, 11(10), 464- 470.
All Contributors
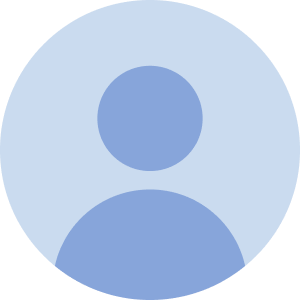
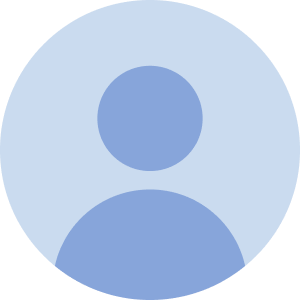
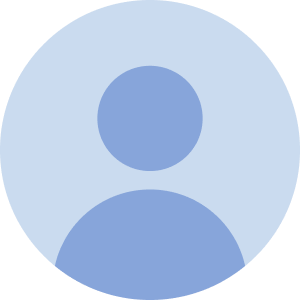